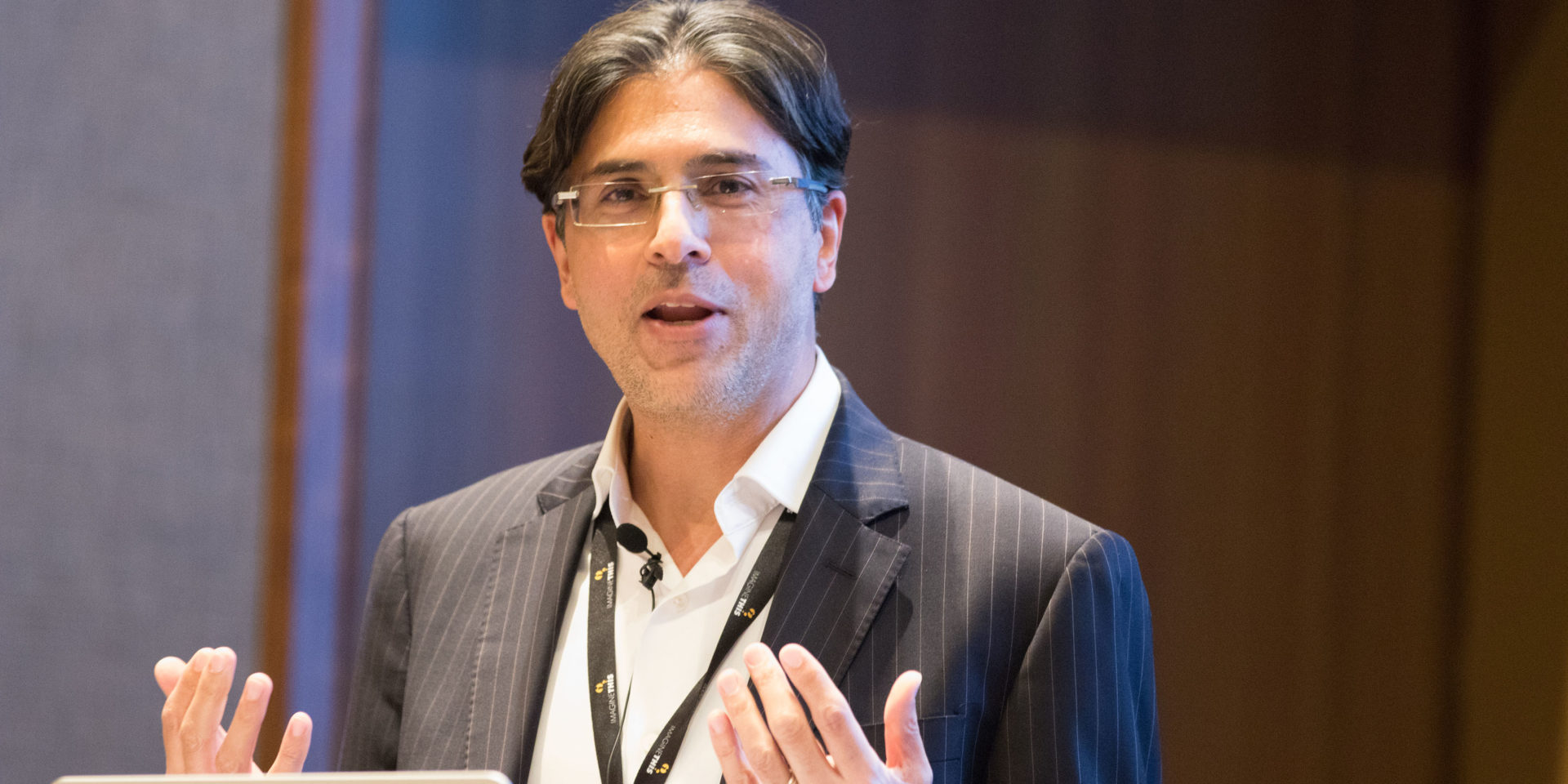
How machine learning will change gaming
Hidden away inside your data are incredible secrets. With lots of data there can be many multiples of insights locked away. But make no mistake – the data holds the answers that you are looking for. You just need to find them.
And with lots of available data, you just need to analyze it in a way that allows you to observe some discernible pattern. It is within those patterns, too complex for you to detect manually, that you will find answers to your most complex problems.
This is exactly what machine learning does: It examines large amounts of data, looking for patterns, and then generates the capability for you to recognize those same patterns in new data. You can use this insight to make better predictions. Put another way, machine learning helps you get smarter with the data that you already have.
For example, suppose that you want to determine with a high degree of confidence, that a promotion will lead to redemption. What’s the right approach for doing this? One option would be to estimate a possible redemption rate for said promotion. The estimate might be based on anecdotal evidence of past conversion, or it may be an artificially created assumption based on your best guess. This approach could work, but it will not be precise.
A different approach would be to look at the available historical data. For example, suppose that you’re trying to find the best approach for detecting offer redemption, and all that you have to work with is the historical redemption data shown in Figure 1.
The good thing about having so little data is that you might be able to find a pattern just by looking at it. The bad thing about having so little data is that the pattern you find is likely to be wrong. Given the data in Figure 1, for example, you might erroneously conclude that males have a higher probability of redemption for this particular promotion.
With more data, your odds of finding a more accurate pattern get better, but finding that pattern will be more difficult. For instance, suppose that you have the set of redemption data shown in Figure 2.
With this much data, it’s immediately obvious that our first guess at a pattern — all males — can’t be right. But if that simple guess was wrong, what’s the right answer? In the data shown here, players older than 55 seem to redeem more. But then look at PlayerId 24552 and 93646 — they don’t fit this pattern. Or is it possible that the offer amount led to the redemption? Even that doesn’t seem right, since both high and low offers were redeemed. Maybe a combination of factors like offer amount and distance to property is fueling redemption. But even this could be suspect.
With some time, you may figure out what is driving redemption, since the data you have to work with isn’t very large. But the permutations could be maddening, even with this small sample size.
What if you had not just the ten records here, but hundreds of thousands of records? And suppose that for each record, you have not only seven columns of data as shown in Figure 2, but 60 columns. There’s probably a useful pattern hidden in that data for determining which players will redeem. But you’ll never figure it out by manually looking at the data – there’s simply too much of it. Instead, you have to use statistical techniques and approaches that are designed for finding patterns in large amounts of data by using a computer and an algorithm.
This is exactly what the machine learning process does. It applies statistical techniques to large amounts of data, looking for the best pattern to solve your problem. It then generates a way for you to recognize that pattern. This is often referred to as a model, and it can be used against your data to solve all types of data-centric problems, like the probability of offer redemption. With our offer redemption problem, a marketer could build a way to detect who to present with an offer, using characteristics from prior redeemed offers. Armed with this information, you could save on promotion costs by only offering to those players who have a high probability of redemption. Or you could evaluate the probability that the gaming behavior associated with the redemption is high, and only offer to those players.
Machine learning plays an important role in this new analytics renaissance. Data wears its secrets as armor, until it’s punctured to reveal the insight. Used in a targeted way, it can unearth insights at immense scale and amazing precision.